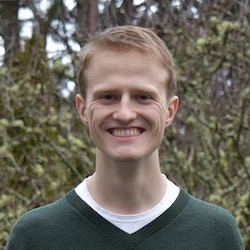
Michael Cooper
Ph.D. Student, Computer Science
University of Toronto
Advised by Rahul G. Krishnan, Michael Brudno
Welcome to my website! I'm Michael - I'm a Ph.D. student in Computer Science at the University of Toronto, where my research focuses on implementing machine learning systems in high-stakes clinical domains like liver transplant prioritization. Motivated by these use cases, I also design and study algorithms to make modern machine learning methods more reliable and interpretable.
Previously, I earned B.S. and M.S. degrees in Computer Science from Stanford University, where I had the privilege of investigating the influence of indoor built space design on human wellbeing with James Landay and Sarah Billington, building an augmented reality application to align patients and medical images in 3D space with Bruce Daniel, and constructing a computer vision dataset comprising complex multi-object multi-actor scenes with Ehsan Adeli and Fei-Fei Li.
In my free time, I enjoy scuba diving, long-distance running, and alpine skiing. I'm also an avid reader of science fiction and historical non-fiction (a few favorites: Liu Cixin's Remembrance of Earth's Past Trilogy, Kim Stanley Robinson's Mars Trilogy, and Ben Rich and Leo James' Skunk Works).
( August 2024 ) Excited to be hosting a workshop on Red Teaming Large Language Models in Healthcare at this year's Machine Learning for Healthcare (MLHC) conference! Come check it out on August 15!
( July 2024 ) Our paper, Predicting Long-Term Allograft Survival in Liver Transplant Recipients, is accepted to Machine Learning for Healthcare (MLHC) 2024!
( June 2024 ) Our paper on interpretable tabular learning has been accepted as a Spotlight Presentation at the International Conference on Machine Learning (ICML) 2024!
- A. Gharari*, M. Cooper*, R. Greiner, RG. Krishnan. "Copula-based Deep Survival Models for Dependent Censoring," Uncertainty in Artificial Intelligence (UAI), 2023.
- M. Cooper*, Z. Ji*, RG. Krishnan. “Machine Learning in Computational Histopathology: Challenges and Opportunities,” Genes, Chromosomes, and Cancer, 2023.
- J. Si, W. Cheng*, M. Cooper*, RG. Krishnan. “InterpreTabNet: Distilling Predictive Signals from Tabular Data by Salient Feature Interpretation,” In International Conference on Machine Learning (ICML), 2024.